The rise of industrial robots is unstoppable. Today, due to their increasingly low cost of operation, they are not only available for large companies, but even SME’s can automate their production (or part of it) with the help of these “smart manipulators”. The popularity of robots has been further fuelled by the recent explosion in collaborative robotics and the breakthroughs in the development of humanoid robots, which are injecting robots even further into consciousness – and into use. Alongside the classic industrial robot manufacturers (Fanuc, KUKA, ABB, Stäubli, etc.), a number of innovative companies (e.g. Universal Robots, Boston Dynamics, etc.) have found their niche at lightning speed and have gained recognition in the industry for their innovative approaches.
Robotic arms are mostly preferred for their manipulation capabilities. In a variety of applications, they can move large masses quickly, accurately and tirelessly. In addition, by integrating appropriate peripherals (e.g. machine vision), they can be made highly intelligent.
With the installation of variously automated production lines, a new area of focus is inevitably added to the companies’ focus: the diagnostics of industrial robots (and manipulators).
Our team has been socialized in an industrial automation and robotics organization, thanks to which we have a deep knowledge of robot control technologies.
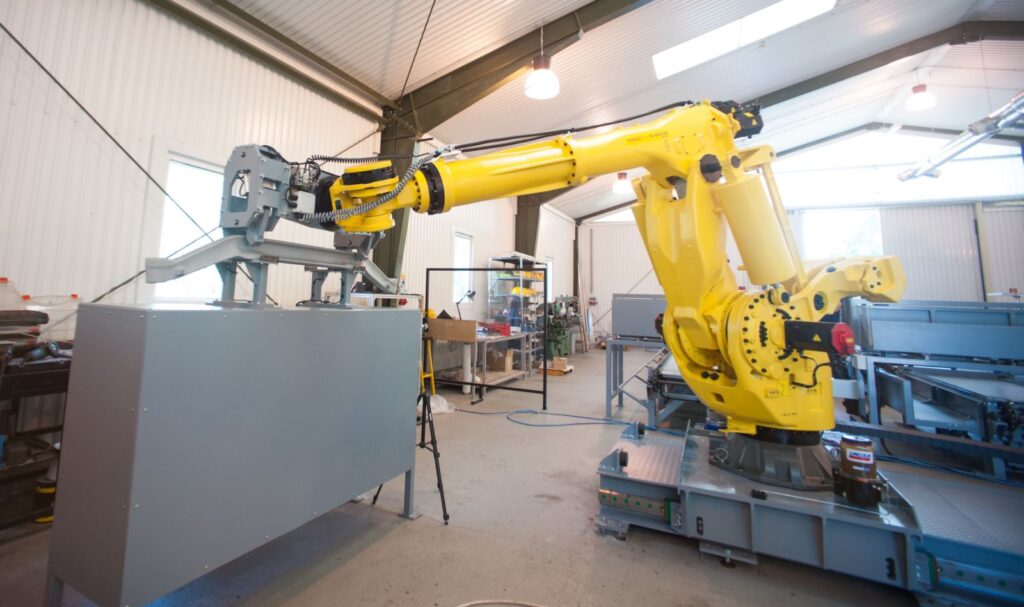
Our strength is developing IIoT projects where we can help our customers by monitoring the parameters and machine data of robotic arms. We have successfully deployed our production monitoring platform WaMeWo for diagnostic tasks, monitoring up to hundreds of robots for our automotive customers.
Maintaining robotic systems
Why does the manufacturing sector use industrial robots? Mainly because of their reliability and predictability. Under the right conditions, these machines can work “for ever”, without stopping. But the right conditions must be created and maintained!
Industrial robotic systems cannot be operated completely maintenance-free over the long term.
The maintenance required may vary from manufacturer to manufacturer, but if the sizing is not ideal, maintenance can easily turn into troubleshooting. And a fault is repaired when the robotic arm is no longer able to function. In a highly robotised environment (e.g. a car manufacturing plant), such a stoppage can generate huge losses within a few minutes, because if a link fails on these production lines, there is typically no plan B (e.g. an operator station) to replace it. In addition, more serious failures cannot be repaired on the densely installed production lines, in which case the complete robot arm is usually replaced.
Although the list of possible faults is endless, there are some typical risks that can be avoided by paying attention to them.
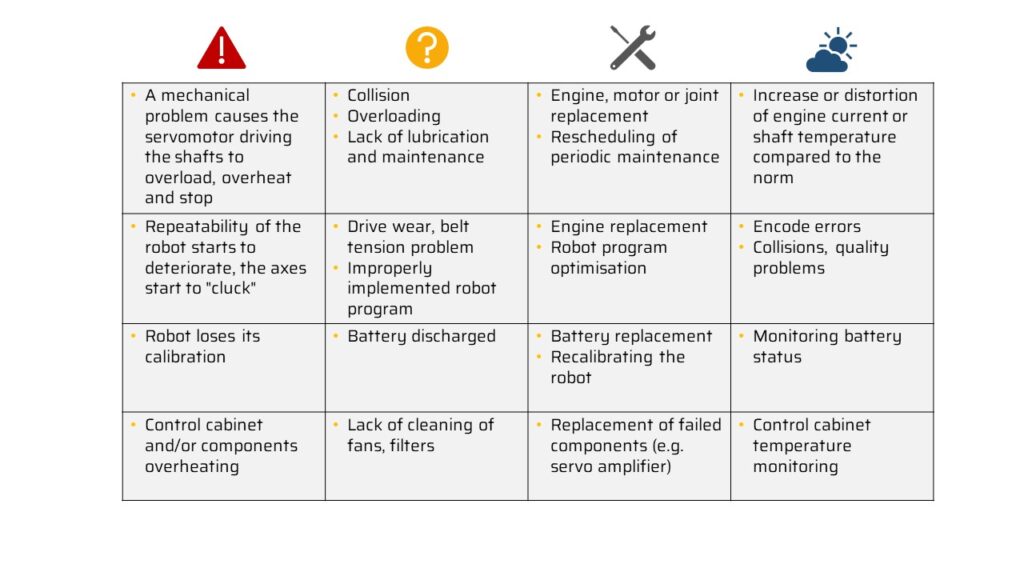
The above examples are (of course) generalisations and there can be drastic differences between robot types.
The common goal of diagnostic testing is to support the work of robotic arm maintainers and optimise the timing of planned maintenance, which can save costs. Some of the data provided by the factory controller can be used to prevent hardware problems with software tools, which typically points towards predictive/preventive maintenance. To forge such benefits, WaMeWo also offers various functionalities.
Case studies
Mechanical diagnostics
With this diagnostic function, you can monitor the various characteristics of controlled actuators with mechanical units (such as robotic arms). The operating parameters are read by WaMeWo directly from the memory of the robot controllers, so no additional sensing or hardware intervention is required. Its most typical application is monitoring of physical parameters of robot arms (e.g. motor currents, temperatures, torques, encoder values, etc. – may vary from robot to robot), which follow a cyclical trend and vary between defined limits during normal operation. If these limits are even approached, exceeded or deviate from the trend, the system will notify subscribed users of the occurrence. Such predictions allow us to be informed of a potential failure before it actually occurs, saving valuable downtime (up to several hours) and optimising planned maintenance cycles.
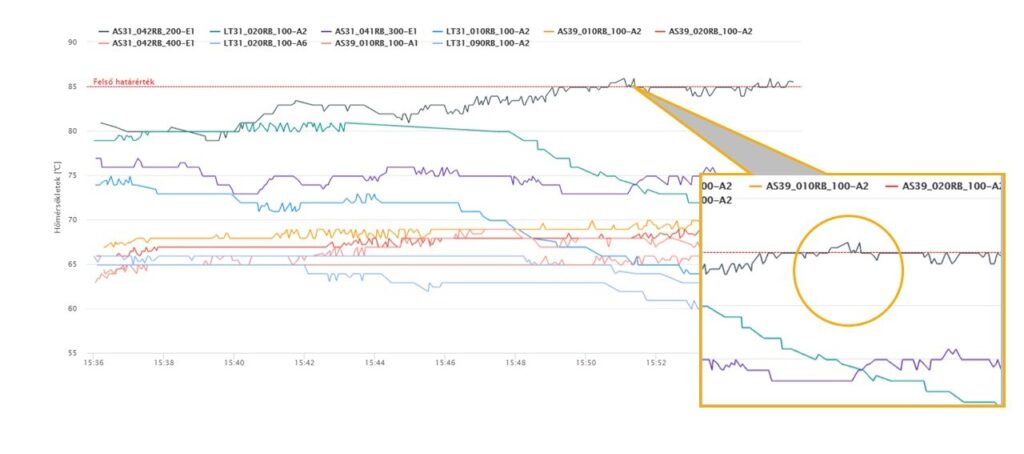
CPU/RAM/HDD monitoring
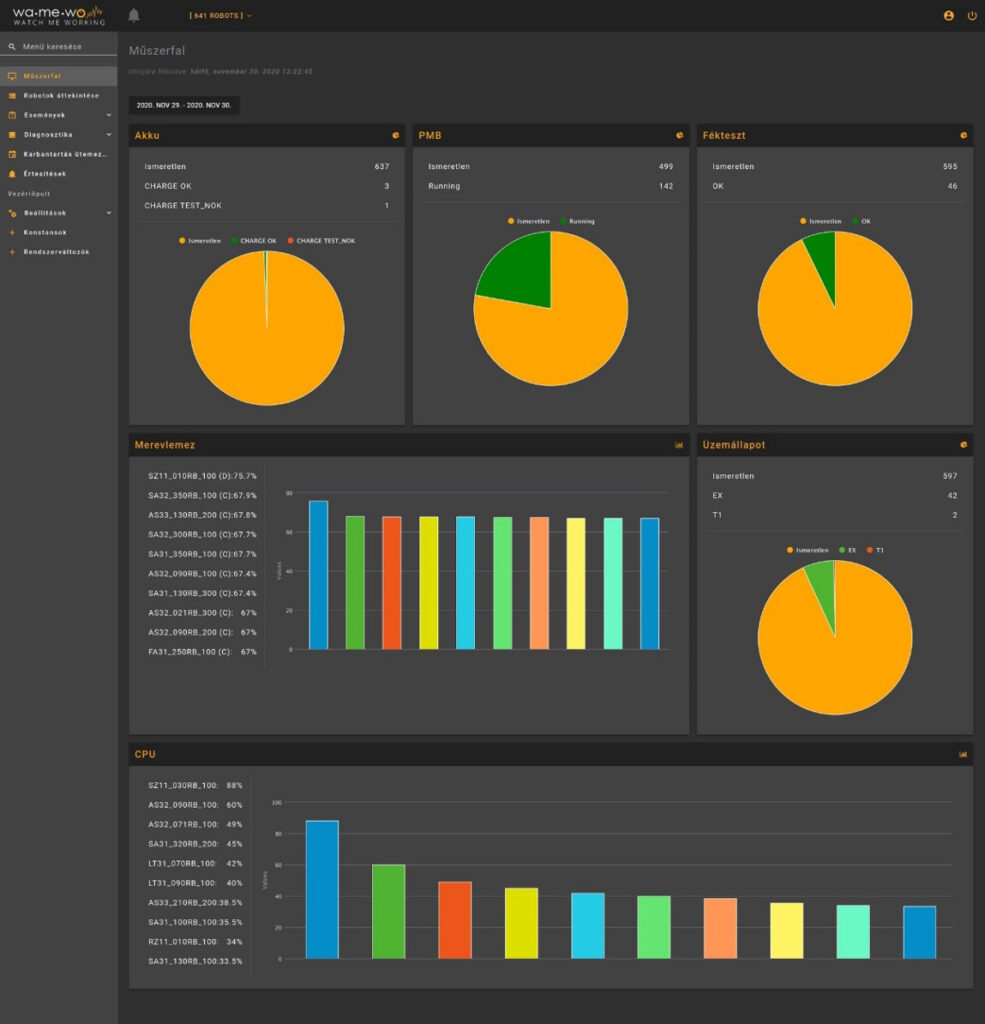
Although most failures occur on the robot arm (as it contains virtually all the moving components), it is also worth monitoring the robot controller. Robot controllers are extremely complex control devices, typically consisting of target hardware, but the necessary computing resources are provided by common components: processor, memory, back-up. Advanced controllers can provide information about these, so that by monitoring them we can filter for controller failure. It is not uncommon for a badly written robot program or software option to overload the CPU, which can cause resource problems for example during robot path generation, and slow down or even stop the robot’s movement.
Battery status
Both earlier generation and modern robots have batteries. Typically (may vary from manufacturer to manufacturer), two sets of batteries are included in the robot system: one in the robot arm and one in the robot controller. In the robot arm, the batteries are necessary to supply power to the encoders (rotary encoders) at the end of the AC servomotors that perform the motions, even when the robot arm is not powered, otherwise calibration problems may occur. And in the case of controllers, permanent power supply is necessary to preserve certain information stored in memory (this is virtually equivalent to the button cell on the motherboard of a PC, which is essential for the BIOS to work).
The exact design of batteries can also vary, but in general it is true that:
- they should be replaced at certain intervals (~yearly) due to their finite lifetime, and
- in a fully unplugged robot, they can only perform their function for a few months, even under ideal conditions.
The importance of maintaining the right battery condition is almost answered by the above examples. If a robot system with a depleted battery is not powered (e.g. in the event of a power failure) and loses its calibration, the robot may become unproductive, which can take hours to correct and verify.
Correlation analysis
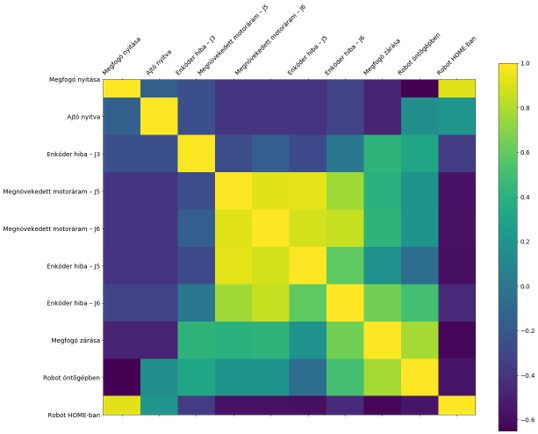
Statistical and predictive methods are based on historic data and cover a wide range of diagnostic possibilities. The temporal and quantitative diagnosis of individual failure events focuses on sequential phenomena. However, it does not address or highlight concurrent events. This is facilitated by the so-called correlation heatmap, a popular tool for investigating correlations between data series. The result of the analysis is strongly influenced by the quantity and quality of the data. By integrating all data sources from the production process into the architecture, complex phenomena can be investigated and solved even on a single analytical interface. For example, a learning algorithm can learn the correlations between tool condition and the number of scrap/shutdown causes, as well as material or operator error, or even tool failure. In the correlation heatmap example below, values close to 1 (in yellow) show a strong positive correlation (correlated over time), while values close to -1 (in blue) show a strong negative correlation (changing over time).
Take aways
A few simple tools can make a huge difference in optimising the maintenance of robotic arms. A well qualified team of experts
use information from the system to prevent unexpected stops or push out the required maintenance window.
At the moment, however, expert competence cannot yet be excluded from this activity: it requires the maintenance staff to receive information, interpret it and physically react (i.e. someone goes to the robot and intervenes to prevent a problem). However, the tools offered by WaMeWo can significantly increase the efficiency of maintenance, as the technicians can arrive much better prepared to deal with an anomaly, making the intervention itself much more targeted, effective and shorter.
And in a more daring vision, in the future, these anomalies will be “self-healed” by machines, or repaired by robots.
What is this series of articles about?
This series of articles has been compiled by our team in order to provide both technological and business know-how for professionals involved in the digitalisation of the industrial sector. The chapters follow two guiding principles:
- exploring the reasons behind the manufacturing digitisation software technologies used and
- demonstrating their potential for business use.
We trust that our content will provoke constructive professional discourse. We welcome your feedback, opinions or questions on info@indeveyes.com-on or our social media platforms!
- In a previous article in this series, we wrote about OEE measurement
- In our next article, we will talk about Customizable, no-code dashboards